The UCL/ICTEAM IEEE Student Branch along with Prof. Ramin Sadre is glad to invite you to the following seminar :
“Cyber Attacks on Internet of Things Sensor Systems for Inference”
given by Prof. Rick S. Blum, professor of Electrical and Computer Engineering at Lehigh University (U.S) and a IEEE Signal Processing Society Distinguished Lecturer.

The event will be held on Monday July 8th 10:00 am in the Shannon meeting room (Maxwell building). The talk will be followed by the presentations of Igor Zavalyshyn, Louai Kechtban and Raziel Carvajal-Gomez, researchers in ICTEAM .
10:00 | Cyber Attacks on Internet of Things Sensor Systems for Inference Rick S. Blum |
11:00 | Coffee Break |
11:15 | IoT Nightmare and How to Deal with It Igor Zavalyshyn |
11:40 | Deep Learning-based device identification using physical wireless signatures Louai Kechtban |
12:05 | Emergent Overlays for Adaptive MANET Broadcast Raziel Carvajal-Gomez |
“Cyber Attacks on Internet of Things Sensor Systems for Inference”
Abstract: The Internet of Things (IoT) improves pervasive sensing and control capabilities via the aid of modern digital communication, signal processing and massive deployment of sensors. The employment of low-cost and spatially distributed IoT sensor nodes with limited hardware and battery power, along with the low required latency to avoid unstable control loops, presents severe security challenges. Attackers can modify the data entering or communicated from the IoT sensors which can have serious impact on any algorithm using this data for inference. In this talk we describe how to provide tight bounds (with sufficient data) on the performance of the best algorithms trying to estimate a parameter from the attacked data and communications under any assumed statistical model describing how the sensor data depends on the parameter before attack. The results hold regardless of the estimation algorithm adopted which could employ deep learning, machine learning, statistical signal processing or any other approach. Example algorithms that achieve performance close to these bounds are illustrated. Attacks that make the attacked data useless for reducing these bounds are also described. These attacks provide a guaranteed attack performance in terms of the bounds regardless of the algorithms the estimation system employs. References are supplied which provide various extensions to all the specific results presented and a brief discussion of applications to IEEE 1588 for clock synchronization is provided.
Biography: Rick S. Blum received a B.S.E.E from Penn State in 1984 and an M.S./Ph.D in EE from the University of Pennsylvania in 1987/1991. From 1984 to 1991 he was with GE Aerospace. Since 1991, he has been at Lehigh University. His research interests include signal processing for cyber security, smart grid, communications, sensor networking, radar and sensor processing. He was an AE for IEEE Trans. on Signal Processing and for IEEE Communications Letters. He has edited special issues for IEEE Trans. on Signal Processing, IEEE Journal of Selected Topics in Signal Processing and IEEE Journal on Selected Areas in Communications. He was a member of the SAM Technical Committee (TC) of the IEEE Signal Processing Society. He was a member of the Signal Processing for Communications TC of the IEEE Signal Processing Society and is a member of the Communications Theory TC of the IEEE Communication Society. He was on the awards Committee of the IEEE Communication Society. Dr. Blum is a Fellow of the IEEE, an IEEE Signal Processing Society Distinguished Lecturer (twice), an IEEE Third Millennium Medal winner, a member of Eta Kappa Nu and Sigma Xi, and holds several patents. He was awarded an ONR Young Investigator Award and an NSFResearch Initiation Award.
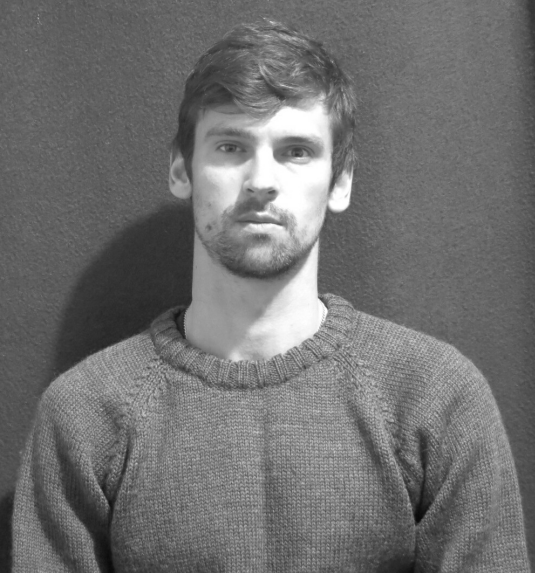
“IoT Nightmare and How to Deal with It”
Abstract: A widespread adoption of Internet-connected devices that can sense and react to changes in the surrounding environment has a tremendous impact on our lives. So-called Internet of Things (IoT) helps to reduce the utility bills, improve the quality of life and cut the production costs. IoT provided a lot of new opportunities in many areas, from healthcare to agriculture, but at the same time, like any other technology, it introduced many challenges.
The number of connected devices and the tremendous amount of data they collectively generate can be overwhelming.
Thus secure and privacy-aware data collection, transfer and processing can be a challenging task. Existing IoT-based systems failed to address this challenge and became the primary target for attacks. Various security vulnerabilities of such systems can lead to profit loss, privacy violation and even death (e.g. health care systems in medical centers). At the same time, some of these systems may be intentionally configured to invade the personal life of their users and gain unauthorized access to sensitive information. We aim to address the security and privacy challenges IoT systems face, as well as to provide the users of such systems with more control over their personal IoT data. We developed a system that may be applied to existing commercial and open source IoT systems, and at the same time act as a foundation for privacy-aware IoT systems
built in the future.
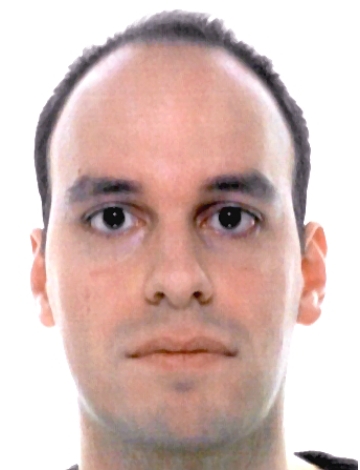
“Deep Learning-based device identification using physical wireless signatures”
Abstract: Device identification using physical wireless signatures can be utilized to assess the identity of a wireless transmitter. Physical characteristics can be mapped onto a large feature space that allows the use of machine learning techniques to accurately discriminate or even identify wireless devices. We propose a deep-learning-based radio identification (DLID) solution that leverages hardware imperfections of internal components of radio transmitters introduced during the manufacturing process to achieve highly accurate device discrimination and identification. We evaluate DLID on an experimental test-bed of 20 identical low-cost WiFi IoT devices. Although our experiments only deal with IEEE 802.11b (2.4 GHz WiFi), our approach could be extended to other wireless communication protocols. The experimental results show that, first, training DLID at different distances from the access point does not reduce the identification accuracy, second, switching transmitting channels has little impact on the identification accuracy, third, DLID can differentiate between network devices with accuracy in excess of 98%.
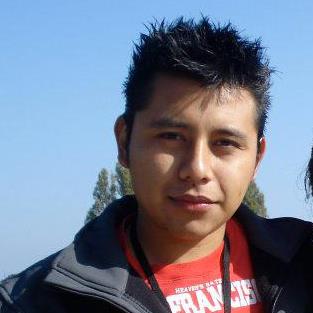
“Emergent Overlays for Adaptive MANET Broadcast”
Abstract : Mobile Ad-Hoc Networks (MANETs) allow distributed applications where no fixed network infrastructure is available. MANETs use wireless communication subject to faults and uncertainty, and must support efficient broadcast. Con- trolled flooding is suitable for highly-dynamic networks, while overlay-based broadcast is suitable for dense and more static ones. Density and mobility vary significantly over a MANET deployment area. We present the design and implementation of emergent overlays for efficient and reliable broadcast in heterogeneous MANETs. This adaptation technique allows nodes to automatically switch from controlled flooding to the use of an overlay. Interoperability protocols support the integration of both protocols in a single heterogeneous system. Coordinated adaptation policies allow regions of nodes to autonomously and collectively emerge and dissolve overlays. Our simulation of the full network stack of 600 mobile nodes shows that emergent overlays reduce energy consumption, and improve reliability and coverage compared to single protocols and to two previously- proposed adaptation techniques.