PPIC : Tool for Sequencial Pattern Mining using CP
The implementations of PPIC performances are presented here. We used six real-life datasets and one synthetic (data200k) with varied characteristics show in Table. 1. Sparsity, representing the average of the number of times items are appeared in each sequence, it is a good indicator to evaluate how sparse or dense dataset is. We can remark then that Kosorak is the largest and the most dense dataset.
Table 1: Dataset Features. Sparsity is equal to (
×∑
)


SDB | #SDB | N | avg(#s) | avg(#I∕s) | max(#s) | sparsity | description |
BIBLE | 36369 | 13905 | 21.64 | 17.85 | 100 | 1.2 | text |
FIFA | 20450 | 2990 | 36.24 | 34.74 | 100 | 1.2 | web click stream |
Kosarak | 69999 | 21144 | 7.98 | 7.98 | 796 | 1.0 | web click stream |
Leviathan | 5834 | 9025 | 33.81 | 26.34 | 100 | 1.3 | text |
PubMed | 17237 | 19931 | 29.56 | 24.82 | 198 | 1.2 | bio-medical text |
data200k | 200000 | 26 | 50.25 | 18.25 | 86 | 2.8 | synthetic data |
protein | 103120 | 25 | 482.25 | 19.93 | 600 | 24.2 | protein sequences |
Our work is carried in OscaR Solver, implemented in Scala and are run under JVM with maximum memory set to 8GB. We used a machine with processor 2.7Hz Intel core i5 and 8GB of RAM with Linux 3.19.0-32-generic 64 bits distribution Mint 17.3. Execution time limit is set to 3600 seconds (1 hour)
.Our proposals are compared with:
- CP-Based Approaches : GapSeq and PP CPSM
- Specialized Approaches : cSPADE spmf.LAPIN PrefixSpan SPAM
- Other : SMA and SPIRIT
- GapSeq: Kemmar, A., Loudni, S., Lebbah, Y., Boizumault, P., Charnois, T.: A global constraint for mining sequential patterns with gap constraint. arXiv preprint arXiv:1511.08350 (2015)
- PP: Kemmar, A., Loudni, S., Lebbah, Y., Boizumault, P., Charnois, T.: Prefixprojection global constraint for sequential pattern mining. In: Principles and Practice of Constraint Programming, Springer (2015) 226–243
- CPSM: Negrevergne, B., Guns, T.: Constraint-based sequence mining using constraint programming. In: Integration of AI and OR Techniques in Constraint Programming. Springer (2015) 288–305
- cSPADE: Zaki, M.J.: Sequence mining in categorical domains: incorporating constraints. In: Proceedings of the ninth international conference on Information and knowledge management, ACM (2000) 422–429
- LAPIN: Yang, Z., Wang, Y., Kitsuregawa, M.: LAPIN: effective sequential pattern mining algorithms by last position induction for dense databases. In: Advances in Databases: Concepts, Systems and Applications, 12th International Conference on Database Systems for Advanced Applications, DASFAA 2007, Bangkok, Thailand, April 9-12, 2007, Proceedings. (2007) 1020–1023
- PrefixSpan: Pei, J., Han, J., Mortazavi-Asl, B., Pinto, H., Chen, Q., Dayal, U., Hsu, M.C.: Prefixspan: Mining sequential patterns efficiently by prefix-projected pattern growth. In: icccn, IEEE (2001) 0215
- SPAM: Ayres, J., Flannick, J., Gehrke, J., Yiu, T.: Sequential pattern mining using a bitmap representation. In: Proceedings of the Eighth ACM SIGKDD International Conference on Knowledge Discovery and Data Mining, July 23-26, 2002, Edmonton, Alberta, Canada. (2002) 429–435
- SMA: Trasarti, R., Bonchi, F., Goethals, B.: Sequence mining automata: A new technique for mining frequent sequences under regular expressions. In: Data Mining, 2008. ICDM’08. Eighth IEEE International Conference on, IEEE (2008) 1061–1066
- SPIRIT : Garofalakis, M.N., Rastogi, R., Shim, K.: Spirit: Sequential pattern mining with regular expression constraints. In: VLDB. Volume 99. (1999) 7–10
PPIC (PPIC, PPDC, PPmixed) vs CP-based approaches
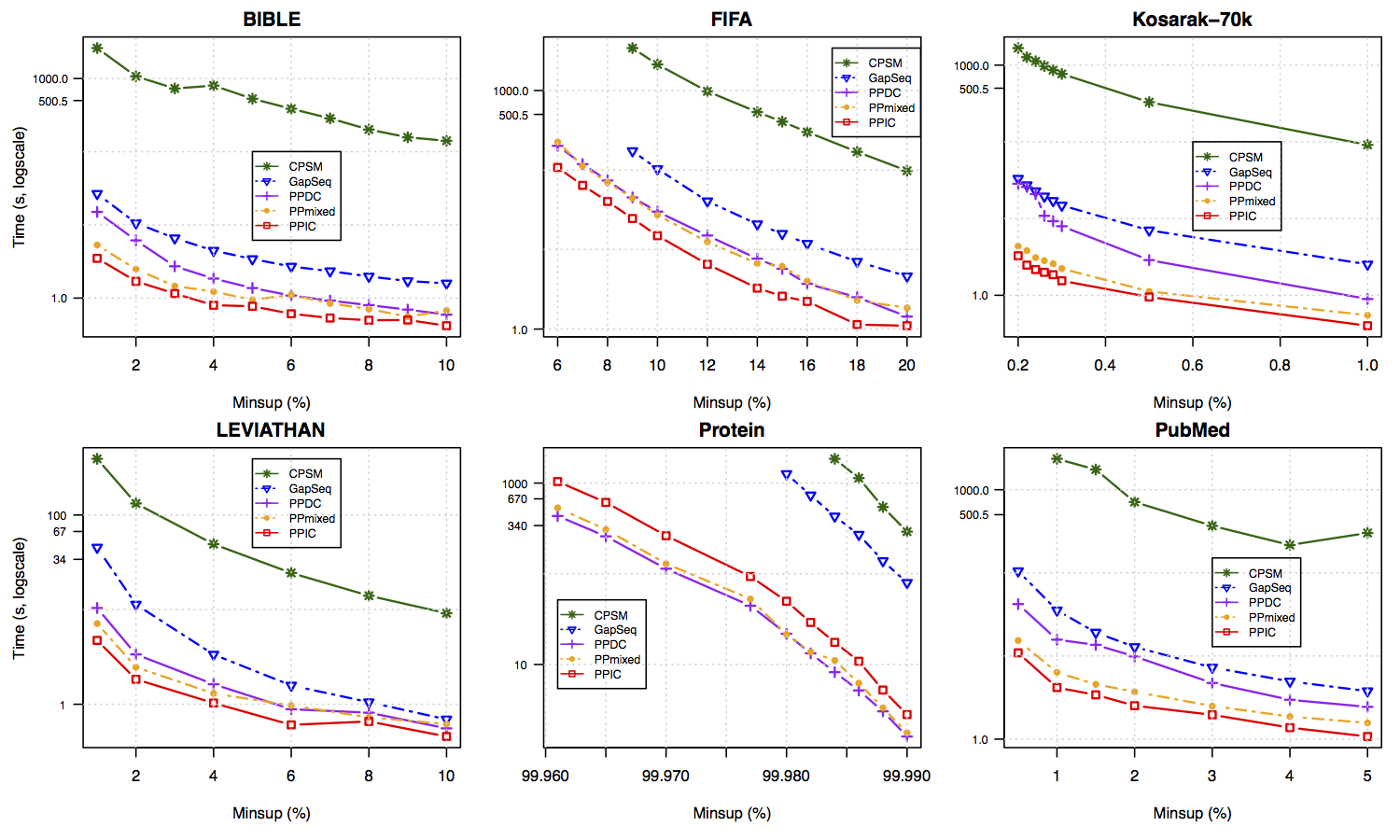
PPIC (PPIC) vs Specialized approaches
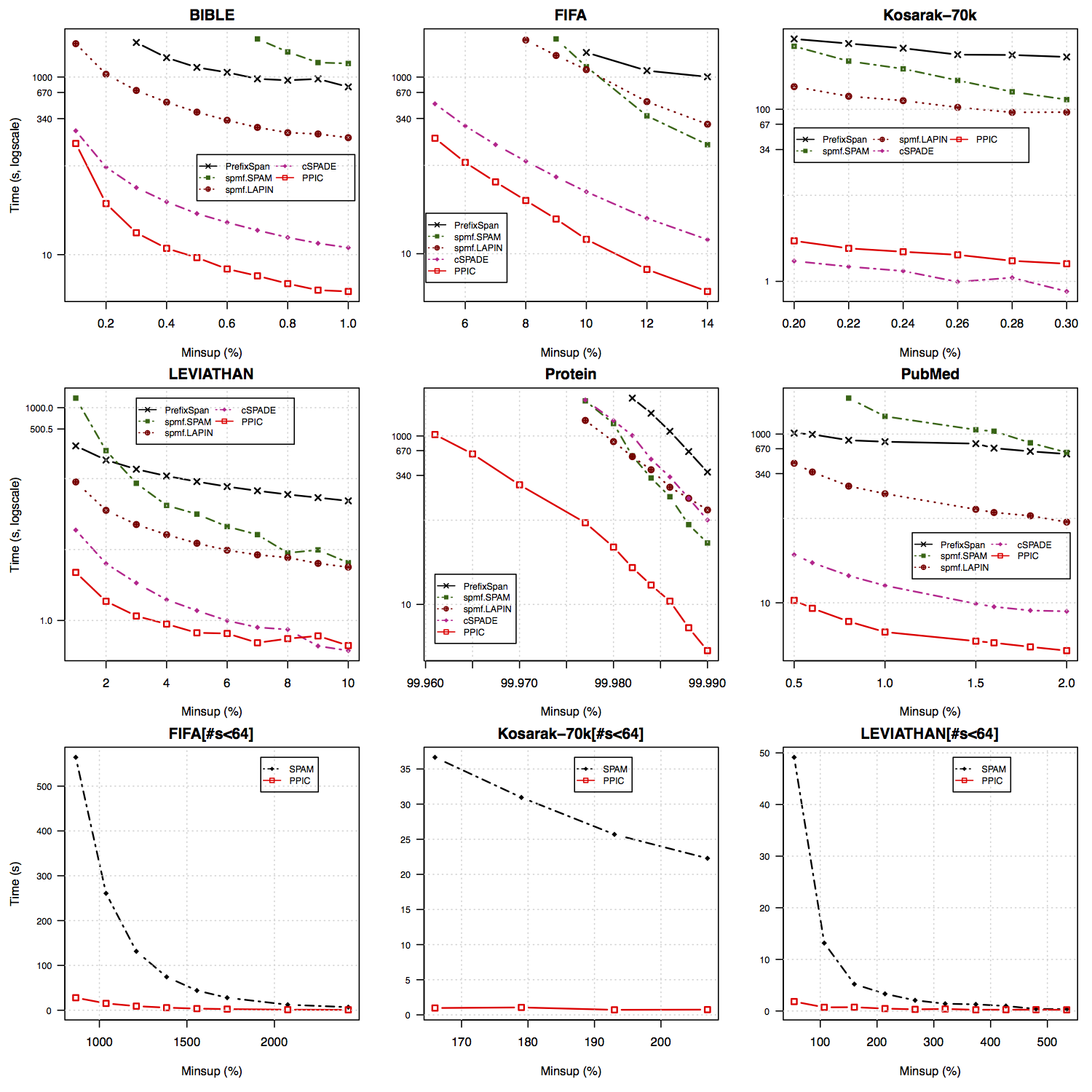
Handling some constraints
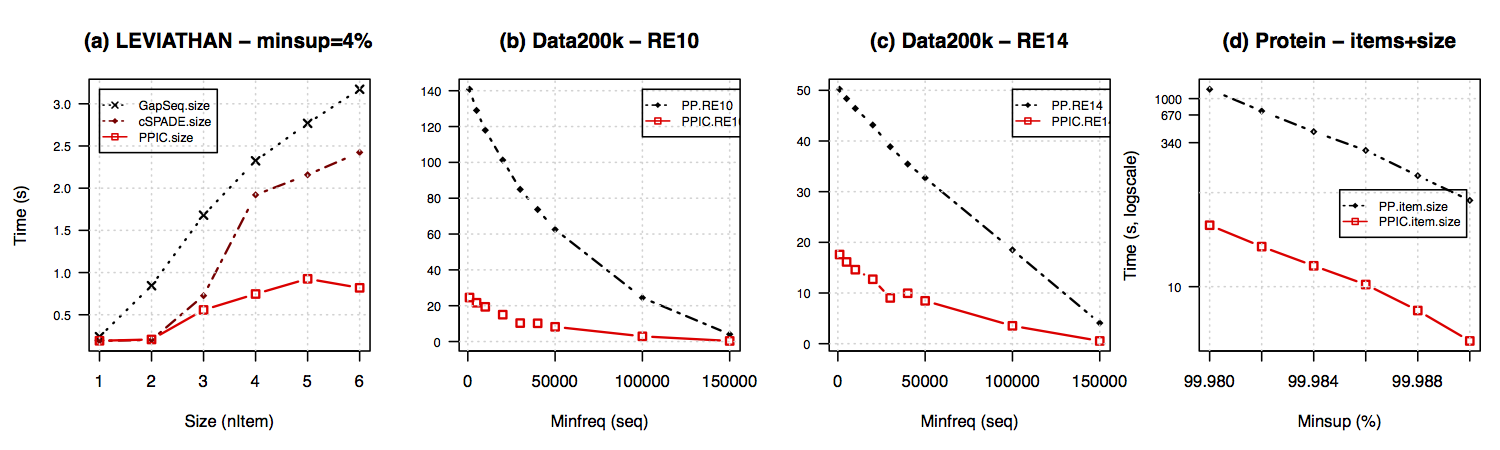