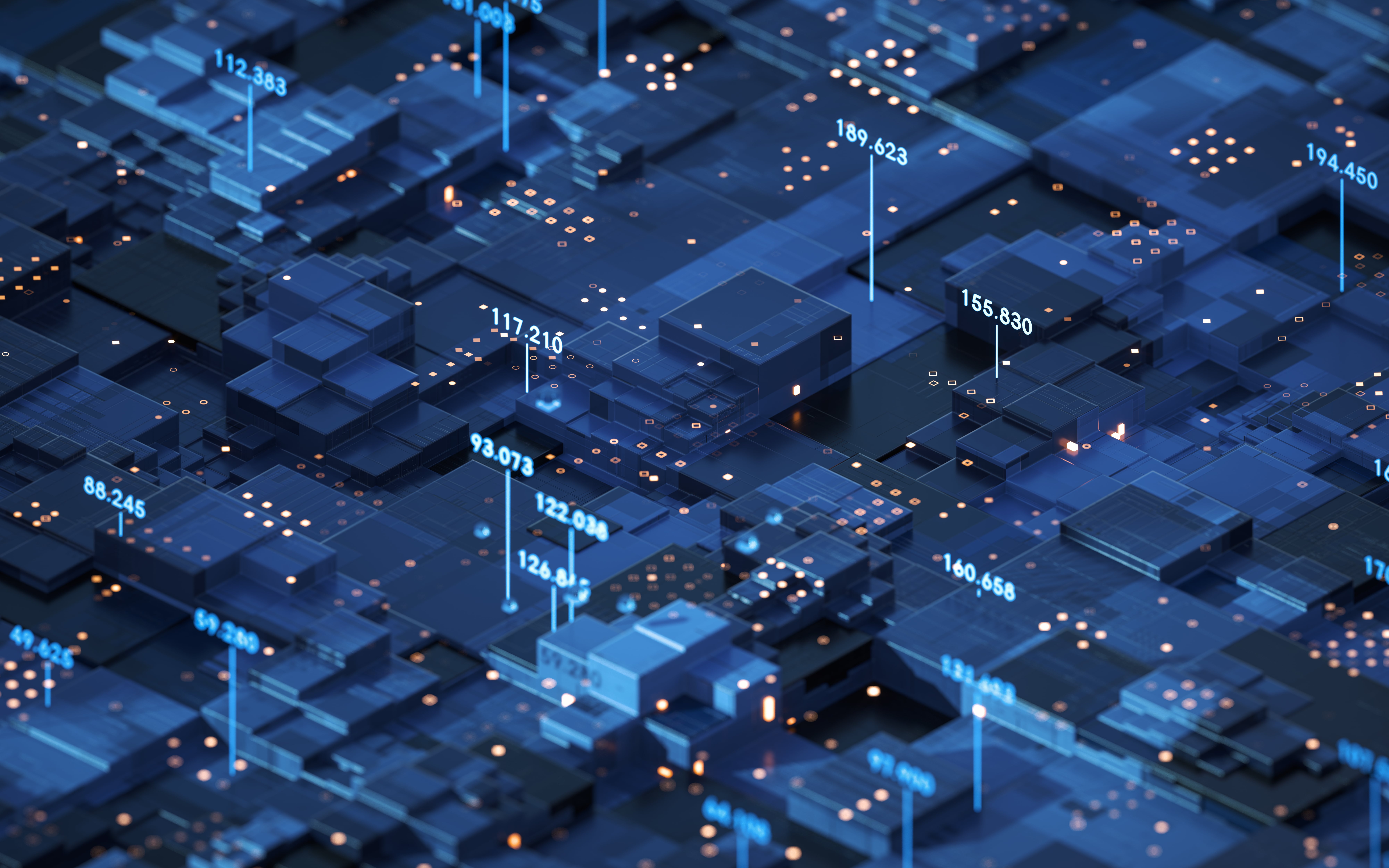
AIDE.
Federated learning enables multiple clients to train a learning model collaboratively in a distributed way. The process comprises the following three steps: (1) local models are learned from local data and algorithms, (2) then aggregated into more comprehensive global models that respect the sharing policies of the participating entities, and (3) finally, the global models are deployed in the infrastructure of the participating entities.
Federated learning, therefore, provides an integrated approach to sharing and learning from information without the need to share massive amounts of sensitive data in a centralized server. This brings substantial advantages in terms of security, confidentiality, and privacy. Federated learning is also more resource-efficient, as it replaces the sharing of huge datasets with the sharing of model parameters.
Systems must be carefully assessed for potential vulnerabilities, and experts must develop appropriate risk-based countermeasures to create robust federated learning systems.
Federated learning, with its wide range of applications, is at the heart of the AIDE project. We are commencing with two practical cases: one in cybersecurity, where the sharing of sensitive information is a critical need, and a second in the sphere of Industrial Internet of Things (IoT). In the context of cybersecurity, the AIDE project’s application of federated learning in threat information sharing can revolutionize penetration testing, enhance the synthesis of automatic code repair for vulnerabilities, and optimize malware analysis, threat intelligence, and intrusion detection. In the IoT domain, the AIDE project’s focus on predictive maintenance is not just theoretical, but it underscores the practical benefits it can bring. This emphasis on practical benefits is sure to resonate with potential partners in the cybersecurity and IoT fields.
Our team and the advisory board can develop further corporate case studies based on the partner network and user group. This endeavor holds significant promise, particularly in the realm of the European digital identity. With the support of personal digital wallets, we can harness privacy-sensitive information that is available locally. The robust, privacy-friendly federated learning that we propose has the potential to open up new and exciting opportunities. Similarly, the SolidLab Flanders project creates an opportunity for federated learning on user data, a prospect that could revolutionize our approach.
We will deploy a demonstrator in the POD (Place of Digital). This demonstrator will be accessible to all socio-economic players wishing to benefit from the advantages of federated learning. The AIDE federated learning platform will enable automated deployment of the federated learning process in several organizations.